Half‑time seminar with Samuel Willingham
Welcome to a half-time seminar with Samuel Willinghamm an Early Stage Researcher in the Plenoptima project, an European H2020 Marie Sklodowska-Curie Innovative Training Network.
Samuel Willingham is an Early Stage Researcher in the Plenoptima project, an European H2020 Marie Sklodowska-Curie Innovative Training Network, a cross-disciplinary collaboration on plenoptic imaging. Samuel is part of a double doctoral degree between Mid Sweden University and INRIA/University of Rennes
Title: Learning Methods for Inverse Problems on Novel Imaging Modalities
Respondent: Samuel Willingham
Opponent: Dr. Luca Calatroni, Laboratoire d'informatique, signaux et systèmes de Sophia-Antipolis (I3S - CNRS/Université Côte d'Azur).
Supervisors: Prof Mårten Sjöström, Mid Sweden University and Director of Research Dr Christine Guillemot, INRIA.
Places:
- Mid Sweden University, Campus Sundsval, room L401
- INRIA: Will be annnounced
- Zoom: Zoom Meeting Link
Abstract
When capturing or processing images, one often encounters noise and other degradations. The challenge of trying to recover a high quality image from a degraded and noisy observation is referred to as an inverse problem. This could refer to completing images with missing pixels, super-resolution or other problems. Because these inverse problems are often ill-posed, some kind of regularization is required. Deep neural nets have emerged as powerful tools for regularization and can be considered state of the art whenever sufficient training-data is available. The aim of this work is to improve the understanding of image regularization and extend existing approaches for 2D images to light fields. This is done by investigating the use of deep neural nets for the solution of inverse problems and further examining how regularizations for 2D inverse problems compare to diffusion models, which are used to generate new images from a data distribution and how both approaches can benefit from one another. These approaches on 2D images will then be extended to light fields. Overall, this work is intended to investigate how deep neural nets can be trained to reconstruct high quality images by leveraging the knowledge of the inverse problem at hand as well as available data.
Contacts
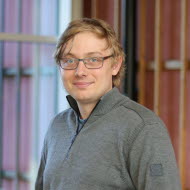